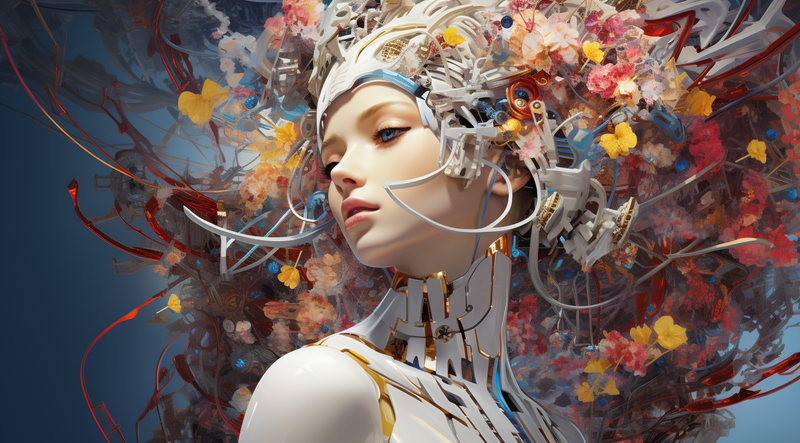
Exploring the World of AI Art: Techniques and Creations
In the ever-evolving landscape of technology, artificial intelligence has found its way into the world of art, creating innovative and fascinating pieces that challenge the traditional boundaries of creativity. AI art techniques are transforming the way artists and enthusiasts approach their craft, offering a myriad of possibilities for self-expression and visual storytelling. In this blog post, we will explore three prominent AI art techniques: style transfer, generative adversarial networks (GANs), and deep learning. We will provide step-by-step guides and showcase examples of how AI is redefining the art world.
AI Art Technique 1: Style Transfer
Style transfer is a remarkable AI art technique that combines the content of one image with the artistic style of another, resulting in a unique and captivating fusion. This technique leverages deep neural networks, particularly Convolutional Neural Networks (CNNs), to analyze and recreate images. Here's how it works:
Preparation: To get started with style transfer, you'll need a content image and a style image. The content image is the subject you want to stylize, and the style image is the artistic reference.
Choose a Pretrained Model: Select a pretrained model like VGG16 or VGG19, which has proven effective for style transfer.
Feature Extraction: Use the CNN to extract features from both the content and style images.
Define Loss Functions: Create loss functions to minimize the difference between the content features and the style features. This guides the algorithm in achieving the desired artistic style while retaining the content.
Optimization: Optimize the content image iteratively to minimize the loss functions and produce the final stylized image.
Example: Consider a photograph of a cityscape (content image) and a famous artwork like Van Gogh's "Starry Night" (style image). By applying style transfer, the cityscape can be transformed to emulate Van Gogh's distinctive style, resulting in a captivating blend of reality and artistic expression.
AI Art Technique 2: Generative Adversarial Networks (GANs)
Generative Adversarial Networks (GANs) are a powerful class of AI models that consist of two neural networks: a generator and a discriminator. GANs are particularly known for their ability to create entirely new and original content. Here's how GANs work:
Training: GANs are trained on a dataset of images. The generator creates new images, while the discriminator tries to distinguish between real and generated images.
Feedback Loop: The generator improves its output over time to fool the discriminator, and the discriminator becomes better at distinguishing real from fake.
Creation: Once trained, GANs can generate images that are often indistinguishable from human-made art. They can produce paintings, photographs, and even entirely new styles.
Example: Artists and developers have used GANs to generate everything from portraits of imaginary people to unique abstract art. For instance, the "Portrait of Edmond de Belamy" by the art collective Obvious is a striking example of GAN-generated art.
AI Art Technique 3: Deep Learning
Deep learning is a fundamental AI technology that underpins various AI art techniques, enabling the creation of art through neural networks with multiple layers. Here's a simplified guide:
Data Collection: Gather a diverse dataset of images relevant to your creative project.
Model Selection: Choose a deep learning architecture like Convolutional Neural Networks (CNNs) for image-based tasks or Recurrent Neural Networks (RNNs) for sequential data.
Training: Train your deep learning model by feeding it the dataset and allowing it to learn patterns, styles, and features.
Generation: The trained model can now generate art based on the patterns it has learned from the training data.
Example: The "AI Dungeon" project by OpenAI uses deep learning to generate interactive, text-based adventures. Users input a scenario, and the model generates a story in response, showcasing the creative potential of AI in storytelling.
Sources
"A Neural Algorithm of Artistic Style" by Leon A. Gatys, et al.
"Generative Adversarial Nets" by Ian Goodfellow, et al.
OpenAI's "AI Dungeon" project.